IOT enabled Insect detection System
Pest monitoring is essential for sustainable farming. Wide monitoring coverage can support farmers in maintaining abundant harvests while pesticides are reduced and used more efficiently. Various research projects studied the use of Internet of Things (IoT) devices to establish smart farming. These devices can gather a vast amount of data to assist the detection of pests. However, when working on remote fields many constraints such as limited cellular coverage and absence of power supply exist. Thus, high computational tasks cannot natively be performed on the fields. To overcome some of these constraints, an embedded camera-based trap system will be developed to detect and identify relevant insect species in our farms and orchards.
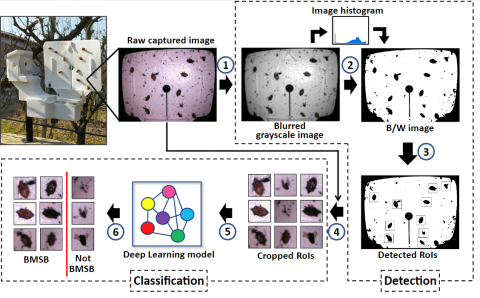
With recent advances in wireless communications, Machine Learning and image recognition algorithms , research is evolving in detecting pests by visual sensors attached on resource constrained edge computing devices for use in the internet of things ( such as a Raspberry Pi ) This project aims to develop a new Low power consumption IoT system for use in remote deployments capable of autonomous behaviour and the identification and population estimation for insect species which are detrimental to harvest production. New edge based image analysis algorithims run on the system and low power consumption communication technologies will inform the farmers as to the information they need to run their farm in the most optimum fashion with the real time data as to the insect population on their farms and orchards. The system will be developed to run for extended periods in remote areas with minimal maintenance.
Thorough pest monitoring is essential for sustainable farming. Wide monitoring coverage can support farmers in maintaining abundant harvests while pesticides are reduced and used more efficiently. Various research projects studied the use of Internet of Things (IoT) devices to establish smart farming. These devices can gather a vast amount of data to assist the detection of pests. However, when working on remote fields many constraints such as limited cellular coverage and absence of power supply exist. Thus, high computational tasks cannot natively be performed on the fields. To overcome some of these constraints, an embedded camera-based trap system will be developed to detect and identify relevant insect species in our farms and orchards. In addition, in terms of sustainability – Biodiversity and an accurate real time census of our insect population is critical in understanding the sustainability of farming best practices and the health of our farmlands. The use of embedded systems and IoT sensors for agricultural monitoring has already been studied in the literature. Insect monitoring requires different techniques to get an overview of the real-time pest situation in the fields. Pest monitoring mainly consists of a sticky-trap with pheromones attached to it. Traditionally, these traps attract bugs and are occasionally monitored in person by entomologists. This can be a tedious and time-consuming task, especially when working on smaller insects such as the carrot fly.
This device is equipped with a camera board to capture images from a double-sided trap. The camera board is an OpenMV Cam H7 Plus which is a low-power and small board based on a microcontroller (STM32H7) supporting Python scripting that makes deployment simple. The trap has a sticky surface that catches HHs with the help of a particular pheromone lure which only attracts HHs and consequently reduces bycatches. Moreover, a trap-based device was suggested to decrease the background complexity of the captured images. In fact, a white trap was chosen because it contrasts most with the brownish colour of the target insect facilitating the machine learning model training to distinguish the insects.
The device was adjusted to capture 2-megapixel images daily during the night using the provided LED. Timing the system to operate at night allows us to control lighting conditions and eliminate ambient lights and shadows; therefore, the captured images have the same conditions in terms of lighting parameters such as light intensity and brightness. In addition, to capture images from both sides of the trap, a servo motor was built into the device, enabling it to cover both sides of the trap for image capturing.
This device was deployed in a pear orchard in the Emilia Romagna region in northern Italy which was infested with Halyomorpha halys between 2022 and 2023.
This device was deployed in a pear orchard in the Emilia Romagna region in northern Italy which was infested with Halyomorpha halys between 2022 and 2023. Next generation iterations are ongoing with a view to developing a startup company in the area of insect detection and monitoring systems or licensing to existing industry partners